CERIST DL
CERIST Digital Library is the institutional repository of the Algerian Research Centre on Scientific and Technical Information (CERIST). It provides access to the entire production of CERIST in terms of journal and conference papers, technical and research reports, theses, course materials, etc. Within CERIST DL, you can:
- Browse the scientific outputs produced at CERIST by communities, collections, authors, etc.
- Search by: Title, Author, Keywords, Publication date, Submission date, etc.
- View and read the existing items available in the repository database. It should be noted that some items are subject to access restrictions.
- Receive alerts on new items and articles by subscribing to one or more collections.
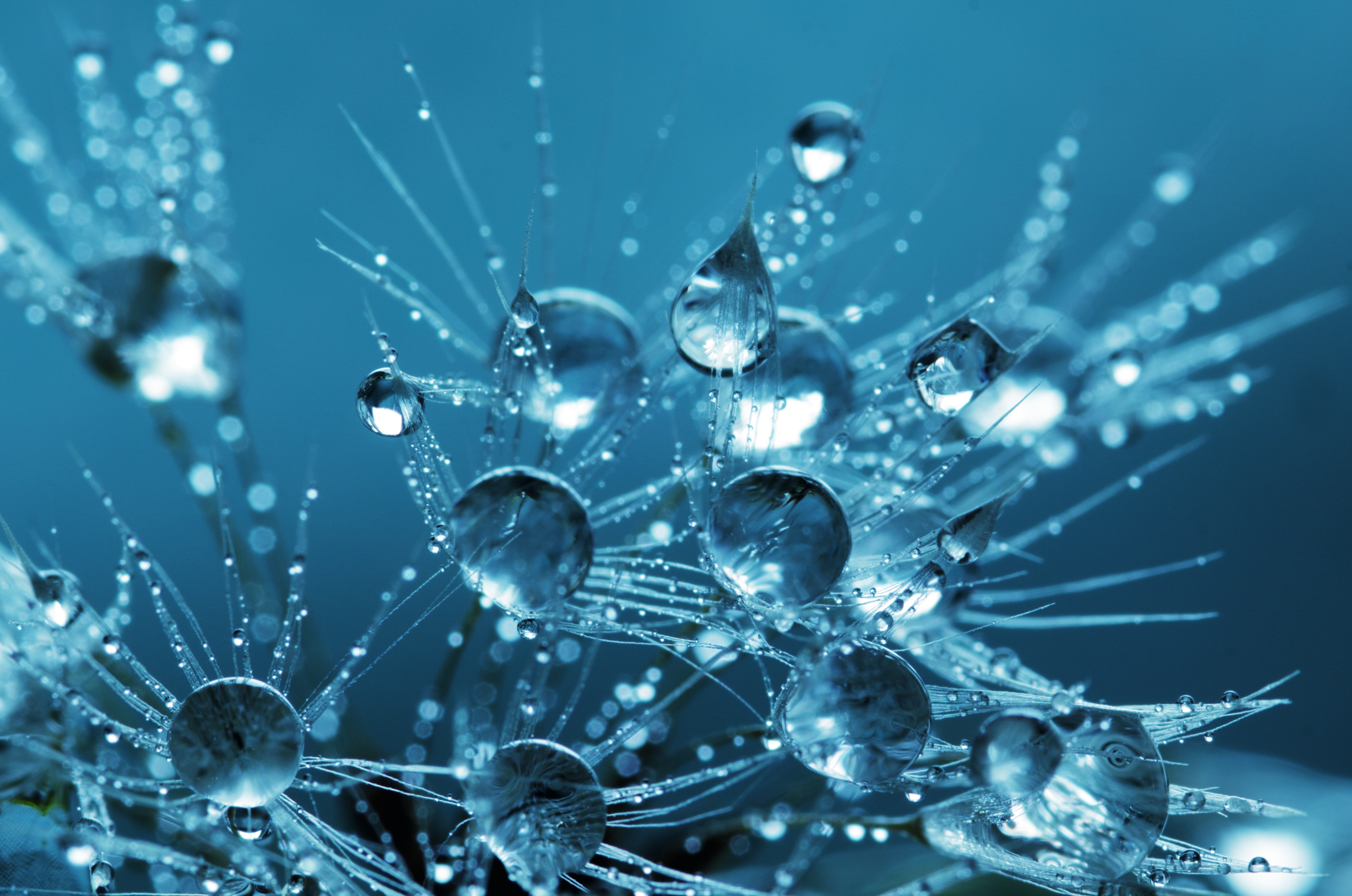
Communities in DSpace
Select a community to browse its collections.
Now showing 1 - 4 of 4
Recent Submissions
Item
Towards Big Data Analytics over Mobile User Data using Machine Learning
(IEEE, 2023-01) Ichou, Sabrina; Hammoudi, Slimane; Cuzzocrea, Alfredo; Meziane, Abdelkrim; Benna, Amel
Machine Learning (ML) is a science that forces computers to learn and behave like humans. As these systems interact with data, networks, and people, they automatically become smarter so that they can eventually solve or predict a practical issue in the world for us. The use of ML can be a giant leap for cannot simply be integrated as the top layer. This requires redefining workflow, architecture, data collection and storage, analytics, and other modules. This paper aims to discuss the issue of machine learning technique for analysis data of mobile user. First, we identified the machine learning benefits and drawbacks, challenges, advantages of using Machine Learning. Then, we propose a generic model of analytic mobile user data using ML, the model is centered on the machine learning component, which interacts with two other components, including mobile user data, and system. The interactions go in both directions. For instance, mobile user data serves as inputs to the learning component and the latter generates outputs; system architecture has impact on how learning algorithms should run and how efficient it is to run them, and simultaneously meeting. Mobile user data goes through several stages: prepossessing which includes the steps we need to follow to transform or encode the data so that it can be easily analyzed by the machine. Then, modelling in this step we will be clustering and classification the data obtained. Finally, evaluation, various measures of performance, accuracy, recall, precision, and F-measure were used to analyze the results of the naive Bayes, SVM, and K-nearest neighbor classification algorithms.
Item
A cooperative framework for automated segmentation of tumors in brain MRI images
(Springer, 2023-03) Hadjadj, Zineb
Brain tumor segmentation from 2D Magnetic Resonance Images (MRI) is an important task for several applications in the field of medical analysis. Commonly, this task is performed manually by medical professionals, but it is not always obvious due to similarities between tumors and normal tissue and variations in tumor appearance. Therefore, the automation of medical image segmentation remains a real challenge that has attracted the attention of several researchers in recent years. Instead of choosing between region and contour approaches, in this article, we propose a region-edge cooperative method for brain tumor segmentation from MRI images. The region approach used is support vector machines (SVMs), one of the popular and highly motivated classification methods, the method distinguishes between normal and abnormal pixels based on some features: intensity and texture. To control and guide the segmentation region, we take advantage of the Ron Kimmel geodesic Active Contour Model (ACM) which produces a good delimitation of the boundaries of the object. The two methods have been cooperated sequentially in order to obtain a flexible and effective system for brain tumor segmentation. Experimental studies are performed on synthetic and real 2D MRI images of various modalities from the radiology unit of the university hospital center in Bab El Oued Algeria. The used MRI images represent various tumor shapes, locations, sizes, and intensities. The proposed cooperative framework outperformed SVM-based segmentation and ACM-based segmentation when executed independently.
Item
Low-cost haptic glove for grasp precision improvement in Virtual Reality-Based Post-Stroke Hand Rehabilitation
(IEEE, ) Masmoudi, Mostefa; Zenati, Nadia; Benbelkacem , Samir; Hadjadj, Zineb
Stroke in Algeria is one of the most important causes of severe physical disability. Upper limb paralysis is also most common in stroke patients, which severely affecting their daily life. Therefore, it is important to help stroke patients to improve the quality of their life. In this article, we have proposed a novel system based on virtual reality for fine motor rehabilitation. Because the sense of touch is essential to the patient's daily activities, we have integrated haptic feedback into our system (vibrating glove), this is to help the patient to perform rehabilitation exercises. The proposed vibrating glove is equipped with five small and flat vibrating motor discs (one on each finger); these motors are controlled by ESP8266 board. This system has been tested on two patients with stroke. The preliminary results show that the system can help patients recover fine motor skills.
Item
Efficient Machine Learning-based Approach for Brain Tumor Detection Using the CAD System
(Taylor & Francis, 2023-04) Guerroudji, Mohamed Amine; Hadjadj, Zineb; Lichouri, Mohamed; Amara, Kahina; Zenati, Nadia
Medical research has focused on improving diagnosis through medical imaging in recent decades. Computer Assisted Diagnosis (CAD) systems have been developed to help doctors identify suspicious areas of interest, particularly those with cancer-like characteristics. CAD systems employ various algorithms and techniques to extract important numerical measurements from medical images that clinicians can use to evaluate patient conditions. This study proposes a statistical classification-based approach to efficient brain cancer detection. The proposed approach operates in three stages: first, Gradient Vector Flow (GVF) Snake models and mathematical morphology techniques retrieve regions of interest. The second stage characterizes these regions using morphological and textural parameters. Finally, a Bayesian network uses this description as input to identify malignant and benign cancer classes. We also compared the performance of the Bayesian network with other popular classification algorithms, including SVM, MLP, KNN, Random Forest, Decision Tree, XGBoost, LGBM, Gaussian Process, and RBF SVM. The results showed the superiority of the Bayesian network for the task of brain tumor classification. The proposed approach has been experimentally validated, with a sensitivity of 100% and a classification accuracy of over 98% for tumors, demonstrating the high efficiency of cancer cell segmentation.
Item
Genetic-Based Algorithm for Task Scheduling in Fog–Cloud Environment
(Springer, ) Khiat, Abdelhamid; Haddadi, Mohamed; Bahnes, Nacera
Over the past few years, there has been a consistent increase in the number of Internet of Things (IoT) devices utilizing Cloud services. However, this growth has brought about new challenges, particularly in terms of latency. To tackle this issue, fog computing has emerged as a promising trend. By incorporating additional resources at the edge of the Cloud architecture, the fog–cloud architecture aims to reduce latency by bringing processing closer to end-users. This trend has significant implications for enhancing the overall performance and user experience of IoT systems. One major challenge in achieving this is minimizing latency without increasing total energy consumption. To address this challenge, it is crucial to employ a powerful scheduling solution. Unfortunately, this scheduling problem is generally known as NP-hard, implying that no optimal solution that can be obtained in a reasonable time has been discovered to date. In this paper, we focus on the problem of task scheduling in a fog–cloud based environment. Therefore, we propose a novel genetic-based algorithm called GAMMR that aims to achieve an optimal balance between total consumed energy and total response time. We evaluate the proposed algorithm using simulations on 8 datasets of varying sizes. The results demonstrate that our proposed GAMMR algorithm outperforms the standard genetic algorithm in all tested cases, with an average improvement of 3.4% in the normalized function.